The Elements of Data Analytic Style
Author: Jeffrey Leek
This book is focused on the details of data analysis that sometimes fall through the cracks in traditional statistics classes and textbooks.
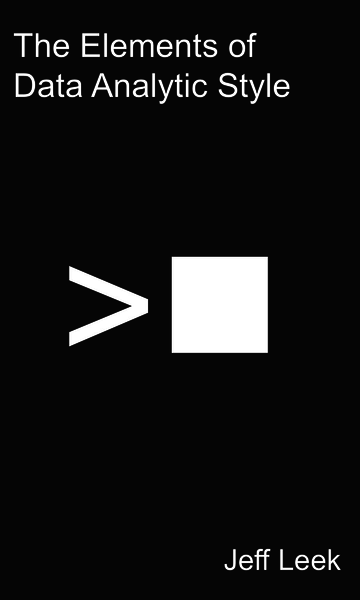
The Art of Data Science
Authors: Roger D. Peng and Elizabeth Matsui
This book writes down the process of data analysis with a minimum of technical detail. What they describe is not a specific "formula" for data analysis, but rather is a general process that can be applied in a variety of situations.
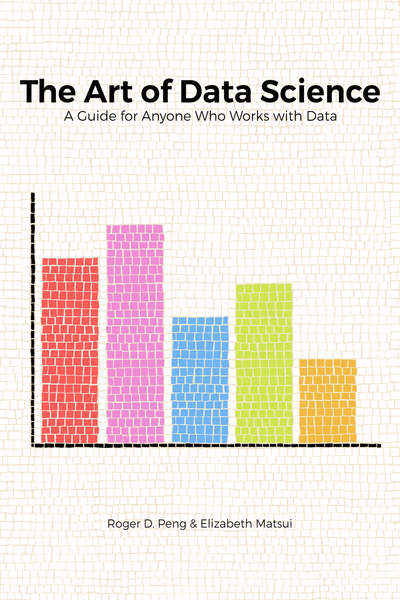
Automate the Boring Stuff With Python
Author: Al Sweigart
In this book, you'll learn how to use Python to write programs that do in minutes what would take you hours to do by hand-no prior programming experience required.
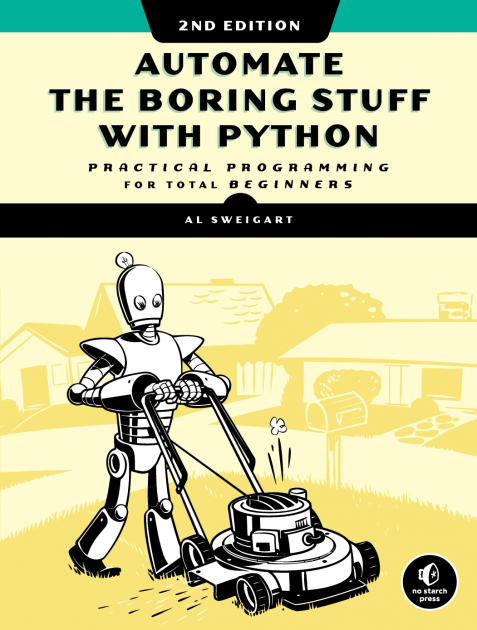
Data Science in Production
Author: Ben G Weber
This book is intended for practitioners that want to get hands-on with building data products across multiple cloud environments and develop skills for applied data science.
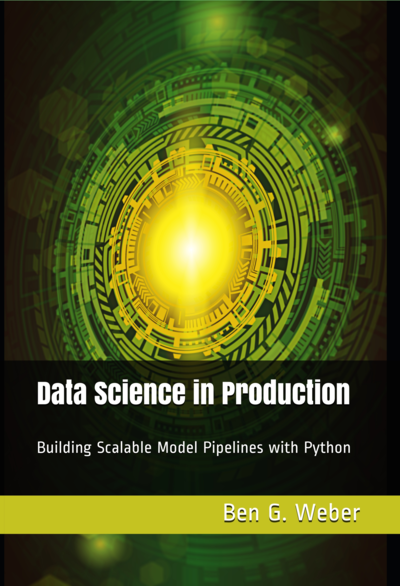
Understanding Machine Learning: From Theory to Algorithms
Authors: Shai Shalev-Shwartz and Shai Ben-David
This book includes a discussion of the computational complexity of learning and the concepts of convexity and stability; important algorithmic paradigms including stochastic gradient descent, neural networks, and structured output learning; and emerging theoretical concepts such as the PAC-Bayes approach and compression-based bounds.
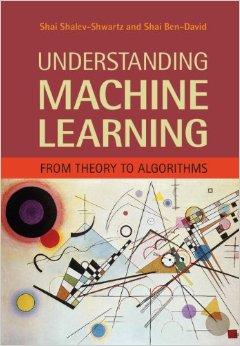
Foundations of Data Science
Authors: Blum, Hopcroft, and Kannan
This data science book is a great blend of lectures in the modern theoretical course in data science.
UFLDL Tutorial
Contributors: Andrew Ng, Jiquan Ngiam, Chuan Yu Foo, Yifan Mai, Caroline Suen

This tutorial aims to get you familiar with the main ideas of Unsupervised Feature Learning and Deep Learning.
Python Data Science Handbook
Author: Jake VanderPlas
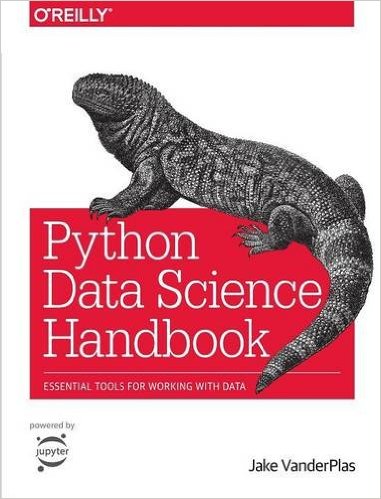
The book introduces the core libraries essential for working with data in Python: particularly IPython, NumPy, Pandas, Matplotlib, Scikit-Learn, and related packages.
Hands-On Machine Learning and Big Data
Authors: Kareem Alkaseer
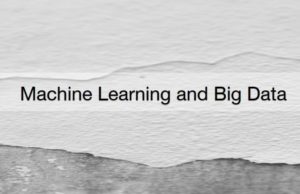
This book is a great source of learning the concepts of Machine Learning and Big Data.
Think Stats
Author: Allen B Downey
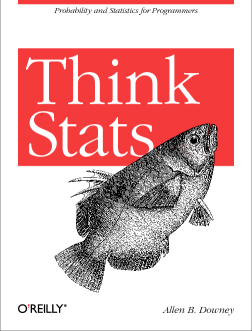
Think Stats emphasizes simple techniques you can use to explore real data sets and answer interesting questions. This one of the most recommended books for data science.
Think Bayes
Author: Allen B Downey
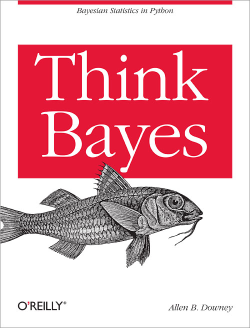
Think Bayes is an introduction to Bayesian statistics using computational methods. This book uses Python code instead of math, and discrete approximations instead of continuous mathematics.
EE263: Introduction to Linear Dynamical Systems
Author: Reza Nasiri Mahalati
This compilation by Professor Sanjay emphasizes on applied linear algebra and linear dynamical systems with applications to circuits, signal processing, communications, and control systems. Link to previous years’ course notes by professor Boyd can be found here.
Convex Optimization – Boyd and Vandenberghe
Author: Stephen Boyd and Lieven Vandenberghe
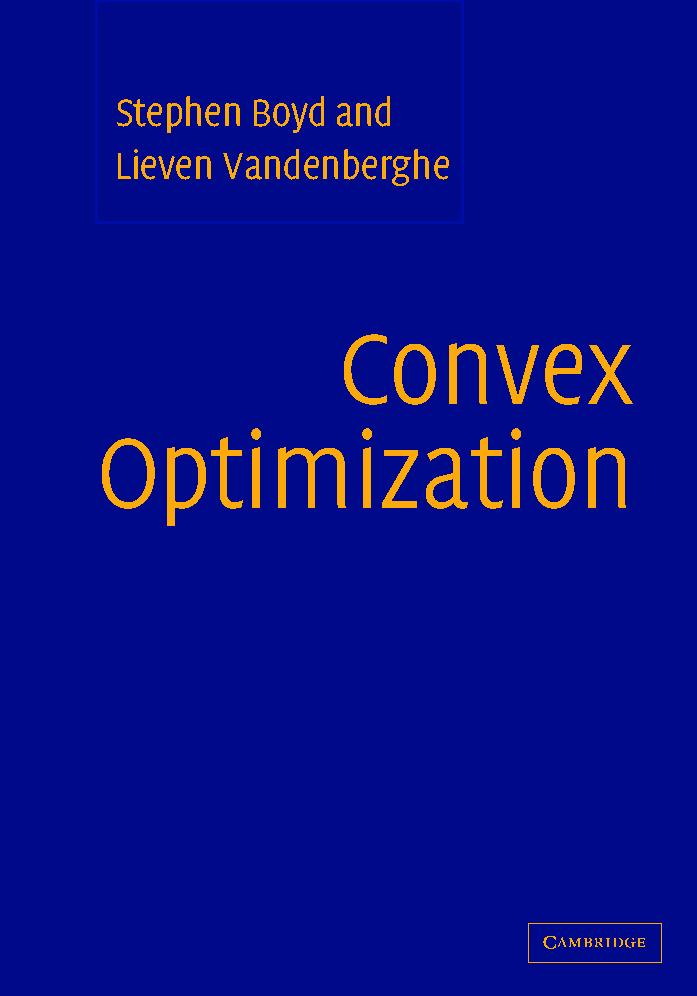
This book provides a comprehensive introduction to the subject and shows in detail how such problems can be solved numerically with great efficiency.
Essentials of Metaheuristics
Author: Sean Luke
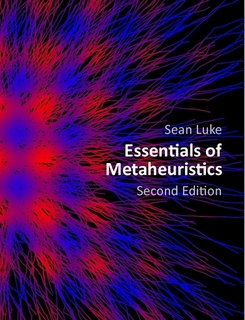
This is an open set of lecture notes on metaheuristics algorithms, intended for undergraduate students, practitioners, programmers, and other non-experts.
CIML
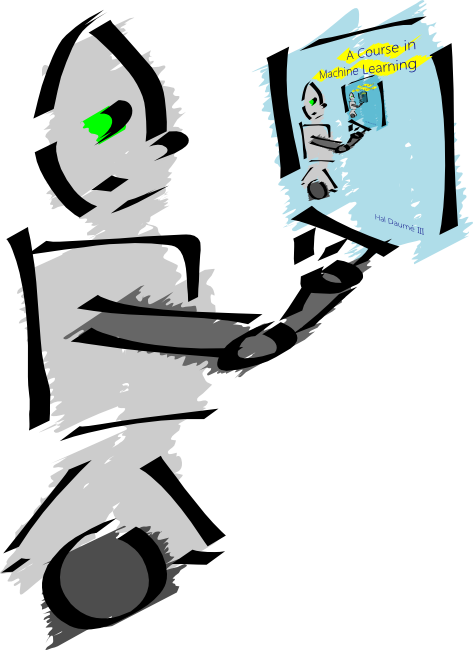
Author: Hal Daumé III
CIML is a set of introductory materials that covers most major aspects of modern machine learning (supervised learning, unsupervised learning, large margin methods, probabilistic modeling, learning theory, etc.).
These are some of